As a tier-1 supplier in the chemical industry, one of the main markets they served was the automotive industry. The manufacturer needed to predict the future demands of their market. By getting insight into the sales volumes and drivers, they would be more equipped to guide their market strategies.
However, this was currently not the case.
What were their challenges
Difficulty forecasting their market share
As a tier-1 supplier in the chemical industry, one of the main markets they served was the automotive industry. They needed to predict the future demands of their market. By getting insight into the sales volumes and drivers, they would be more equipped to guide their market strategies. This was also necessary to help them uncover key demand drivers by industry and region at a quicker pace to stay competitive.
Inability to allocate resources efficiently due to little clarity over market shifts
They were not being able to forecast demand accurately which meant there was an inability to respond to its demand fluctuation, and accurately predict supply on time. The lack of clarity over the impact of external macroeconomic factors meant that they did not have full visibility over resource allocation. This led to unnecessary costs and potential lost revenue.
They needed to identify their leading indicators in order to detect market trend shifts
They had sufficient data which meant that they were a prime candidate to apply multivariate models to their forecasting. However, they were currently using a bottom-up approach, which limited them to using simple univariate forecasting modes. This not only impacted forecasting accuracy, but it also meant that they were missing out on the opportunity to apply leading indicators to their aggregated forecast as univariate forecasting models do not allow for that.
What did they want to achieve?
01 Minimize forecast error margin
02 Identify their leading indicators in order to detect market drivers
03 Uncover key demand drivers by industry and region
The lack of clarity over the impact of external macroeconomic factors meant that they did not have full visibility over resource allocation.
Here were the results:
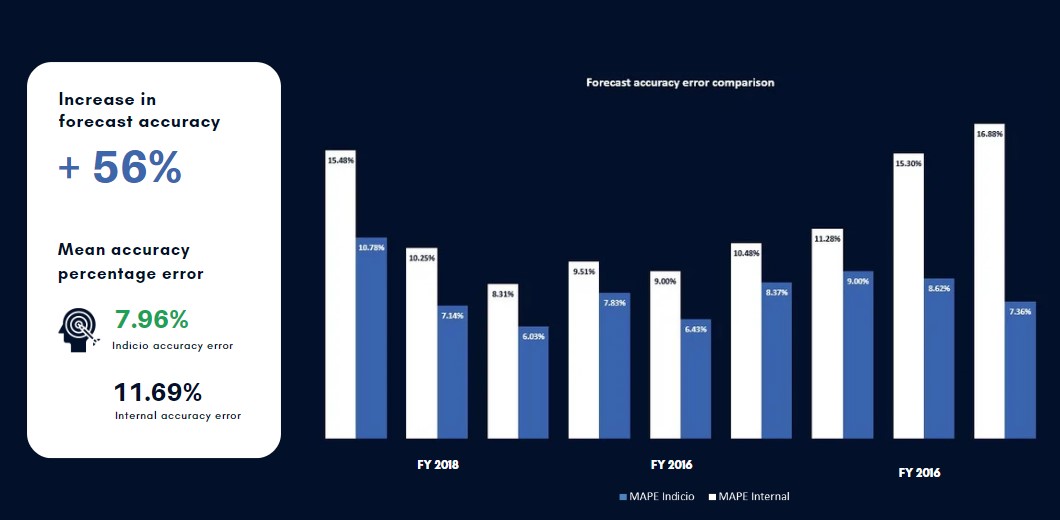
Improved forecast accuracy by 56%
By implementing best practices through all stages of the forecast process, the manufacturer achieved a double-digit MAPE forecast accuracy improvement. When using Indicio to forecast their data, the results were 18-56% more accurate at 2-11 months ahead.
Overview of market drivers at both industry and region levels
The organization was now able to create forecasts easily for each product group and region.
With the models built, it simply requires a quick update monthly, making the process repeatable, saving valuable time.
Ability to track market shifts at a higher frequency
Visibility into the changing relevancy of the organization's leading indicators, which gave them a clearer picture of which inputs to consider.
Download the full case study here.