Limitations of using only univariate models when forecasting. Here are the hidden costs.
1. Not getting the full picture of what’s influencing demand
Univariate models consider only one variable, disregarding the potential influence and interactions of other relevant factors that may influence demand, such as market trends, external events, competitor actions, or economic indicators. By overlooking the broader context, these models may miss crucial information that could significantly impact the accuracy of the forecast. Neglecting external variables and their relationships can lead to incomplete or misleading predictions.
2. Experiencing production inefficiencies which lead to increased operational costs
In industries where production planning is based on demand forecasts, univariate models may fail to provide accurate projections. This can lead to inefficiencies in the production process, such as underutilization of resources during low-demand periods or production bottlenecks during unexpected spikes in demand. These inefficiencies can increase operational costs. For example, having a surplus inventory can also mean that you incur higher carrying costs, including storage fees, insurance, and depreciation, which can impact profitability.
3. Inadequate detection of market trend shifts
Univariate models may struggle to detect and adapt to market trend shifts, particularly in low-volume product scenarios. These models often rely on historical data, assuming that future patterns will mimic the past. However, market dynamics can change rapidly, and univariate models may fail to capture sudden shifts, emerging trends, or disruptive events, resulting in inaccurate forecasts.
4. Overlooking multidimensional insights
Univariate models focus solely on one aspect of the forecasted variable, potentially overlooking multidimensional insights that could be valuable for decision-making. By neglecting the influence of related variables or contextual information, these models may provide limited perspectives on the underlying dynamics driving the forecast. Incorporating additional dimensions through multivariate models allows for a more comprehensive understanding and more robust predictions.
5. Inability to capture interdependencies
In many industries, demand for one product can be influenced by the demand for other related products or services. Univariate models fail to capture these interdependencies, leading to inaccurate forecasts when demand patterns are connected.
Misaligned forecasts can lead to inefficient production planning, resulting in the under-utilization of resources during low-demand periods and bottlenecks during unexpected spikes in demand.
6. Ignoring seasonality and trends
Univariate models may struggle to account for seasonal patterns, such as increased demand during certain time periods or different sales cycles throughout the year. Similarly, they might not effectively capture long-term trends that can impact demand over time.
7. Vulnerability to outliers
Univariate models are more susceptible to outliers or extreme data points. When unusual events occur, such as supply chain disruptions or sudden shifts in customer behavior, these models might provide unreliable forecasts. To compound that, identifying outliers can take quite a bit of time. The upside is that these time-intensive algorithms to identify them do provide more accurate forecast results. Having reliable outlier detection in place ensures you're only dealing with clean data.
8. How much is reactive decision making costing you?
Many demand forecasters find themselves reacting to demand fluctuations, rather than proactively planning or future changes. When using univariate models, you’re not getting sufficient insights into the underlying drivers of demand. The cost? Rush orders, emergency logistics, and missed cost-saving opportunities.
9. Lack of flexibility holding you back from adapting your results to changing market conditions
Univariate models are generally simplistic and lack the flexibility to adapt to changing market conditions or dynamic business environments. More sophisticated multivariate models can better accommodate variations and complexities in demand patterns.
10. Inefficiency when dealing with complex demand patterns:
Univariate models might struggle to capture demand patterns with multiple peaks, irregular fluctuations, or sudden changes. In such cases, more advanced forecasting methods, like machine learning algorithms, can be more effective.
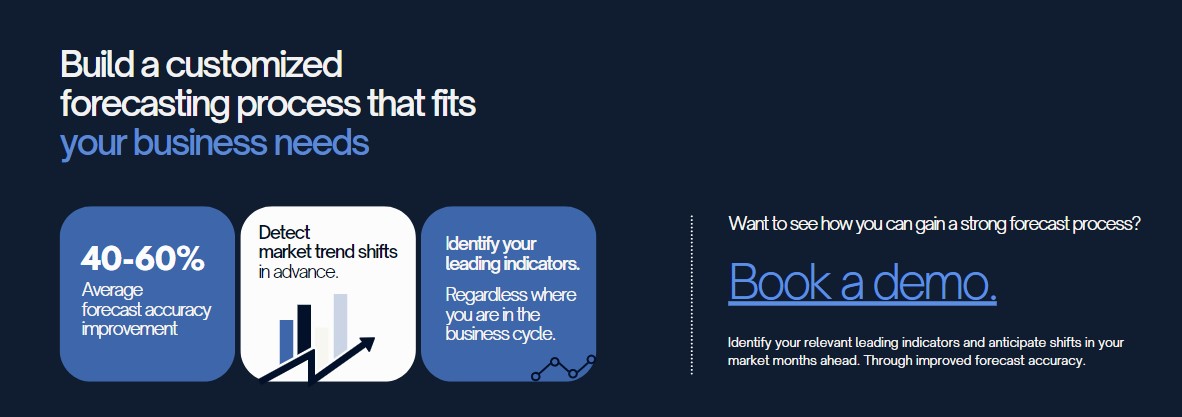